Evolving Data Protection to Keep Up with Modern Needs There was once a time, before privacy concerns and regulations were prevalent, in which sending clear text was the norm – and you may be surprised, but it does still happen today. Rising concern then led to the introduction of encryption, a method which protects data well while in transit – but requires that you give custody of a copy of your data and supply the decryption key to your partner. This makes it fully re-identifiable once the recipient decrypts it and leaves unnecessary, residual data in your partner’s environment; the identities and attributes of individuals that were not common to both you and your partner’s file. Hashing then came into play, scrambling data in a way that’s very difficult to reverse. However, this still leaves data changing custody, which can lead to future re-identification attempts against an identity graph. Also, hashing is still considered identifiable data and therefore isn’t GDPR-compliant, meaning in today’s data ecosystem, it’s still not enough. More recent innovations in privacy-enhancing technology include federated learning, differential privacy, and fully homomorphic encryption. While each is powerful for use in analysis, modeling, and data obfuscation, none of these methods adequately addresses the data linkage problem – a cause for many challenges when it comes to data collaboration. What we’re still missing is the ability to adequately protect PII, while also having a meaningful impact on sharing insights on matched identities. Even data clean rooms, which have become more common today, require that both partners agree on which clean room solution to use and then allow an additional third party to gain custody over all datasets used for matching. It’s a full- sharing event with consent and security obligations, subjecting PII to a level of risk. 10
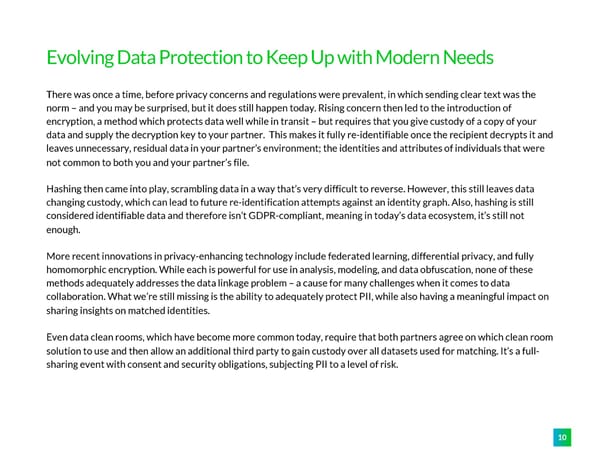